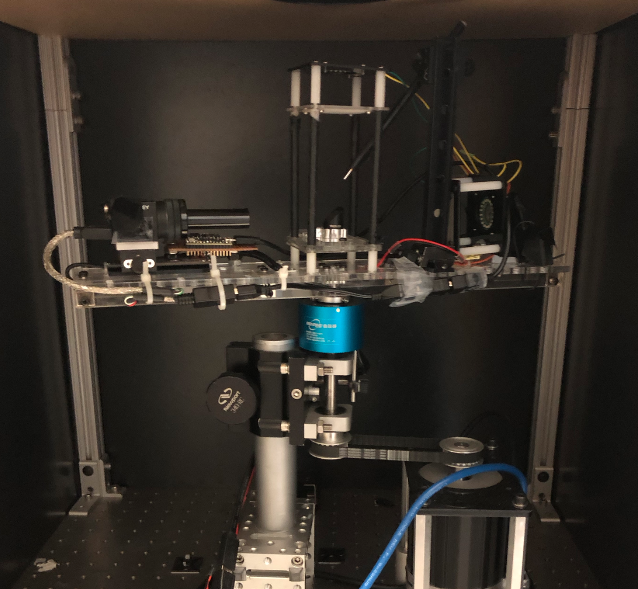
Haltere mediated equilibrium reflex
One of the key requirements for successful flight is the ability to rapidly stabilize to correct for external pertubations. The halteres are structures that are unique to flies and are known to play a critical role in this process. The halteres are hypothesized to detect coriolis forces via multiple fields of strain sensing cells. Using genetic tools, I am currently working on understanding the role of these fields for wing and head stabilization in fruit flies.